AMSGrad
Published on: August 4, 2021
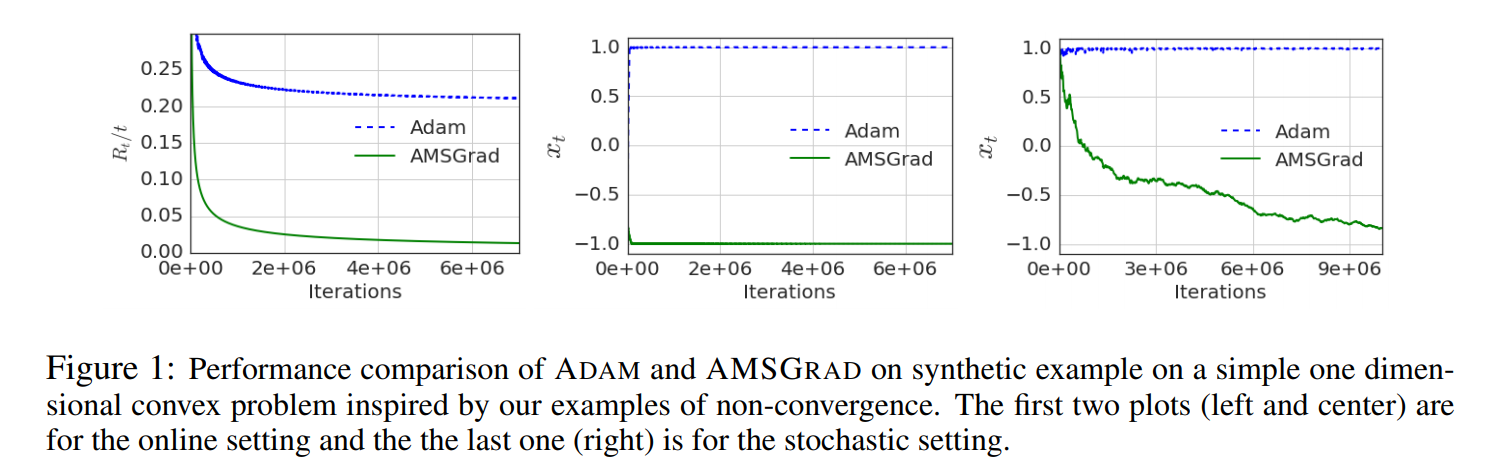
Table of Content
The motivation for AMSGrad lies with the observation that Adam fails to converge to an optimal solution for some data-sets and is outperformed by SDG with momentum.
Reddi et al. (2018) [1] show that one cause of the issue described above is the use of the exponential moving average of the past squared gradients.
To fix the above-described behavior, the authors propose a new algorithm called AMSGrad that keeps a running maximum of the squared gradients instead of an exponential moving average.
For simplicity, the authors also removed the debiasing step, which leads to the following update rule:
For more information, check out the paper 'On the Convergence of Adam and Beyond' and the AMSGrad section of the 'An overview of gradient descent optimization algorithms' article.
[1] Reddi, Sashank J., Kale, Satyen, & Kumar, Sanjiv. [On the Convergence of Adam and Beyond](https://arxiv.org/abs/1904.09237v1).